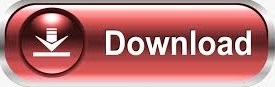
The function can be used in conjunction with any of the usual effect size or outcome measures used in meta-analyses (e.g., as computed using the escalc function). The rma.uni function (with alias rma) provides a general framework for fitting such models. The various meta-analytic models that are typically used in practice are special cases of the general linear (mixed-effects) model. The presence of funnel plot asymmetry (which may be indicative of publication bias) and its potential impact on the results can be examined via the rank correlation and Egger's regression test, the trim and fill method, and by applying a variety of selection models.
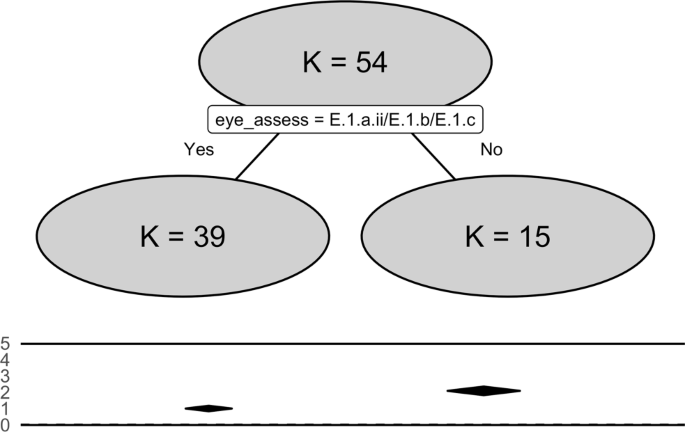
The package also provides functions for creating forest, funnel, radial (Galbraith), normal quantile-quantile, L'Abbé, Baujat, bubble, and GOSH plots. Advanced techniques for hypothesis testing and obtaining confidence intervals (e.g., for the average effect or outcome or for the model coefficients in a meta-regression model) have also been implemented (e.g., the Knapp and Hartung method, permutation tests, cluster robust inference methods / robust variance estimation). Various methods are available to assess model fit, to identify outliers and/or influential studies, and for conducting sensitivity analyses (e.g., standardized residuals, Cook's distances, leave-one-out analyses). For non-independent effects/outcomes (e.g., due to correlated sampling errors, correlated true effects or outcomes, or other forms of clustering), the package also provides a function for fitting multilevel/multivariate meta-analytic models. For meta-analyses of \(2 \times 2\) tables, proportions, incidence rates, and incidence rate ratios, the package also provides functions that implement specialized methods, including the Mantel-Haenszel method, Peto's method, and a variety of suitable generalized linear mixed-effects models (i.e., mixed-effects logistic and Poisson regression models). By including study-level variables (‘moderators’) as predictors in these models, mixed-effects meta-regression models can also be fitted. The package can be used to calculate various effect size or outcome measures and then allows the user to fit fixed- and random-effects models to these data.
33710-5.fp.png)
The metafor package provides a comprehensive collection of functions for conducting meta-analyses in R.
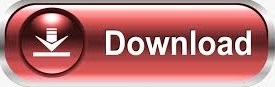